When AI models are trained with a diversity of images curated and labeled by experienced radiologists, they can automatically label new images and suggest diagnoses, which can streamline the workflow of radiologists, enhance accuracy, contribute to training future healthcare professionals, and help analyze vast amounts of data in large clinical studies. During his MSc degree in Mathematics and Statistics, Kevin Raina developed novel methods to aid in detection and delineation of stroke lesions and brain tumors in medical images, a challenging and time-consuming task for radiologists.
The natural bilateral symmetry of a healthy human brain is lost when a patient develops tumors or lesions. In their analysis of Magnetic Resonance (MR) neuroimages, Kevin and his supervisor Professor Tanya Schmah, used symmetry-based information to augment their statistical models for stroke lesion delineation and observed significant improvements in accuracy. Lesion delineation is an example of a more general task called segmentation, in which a digital image is broken down into various regions, or segments, to make further processing or analysis of the image simpler. Taking symmetry into account can potentially improve the quality of neuroimage segmentation and other automated neuroimaging methods.
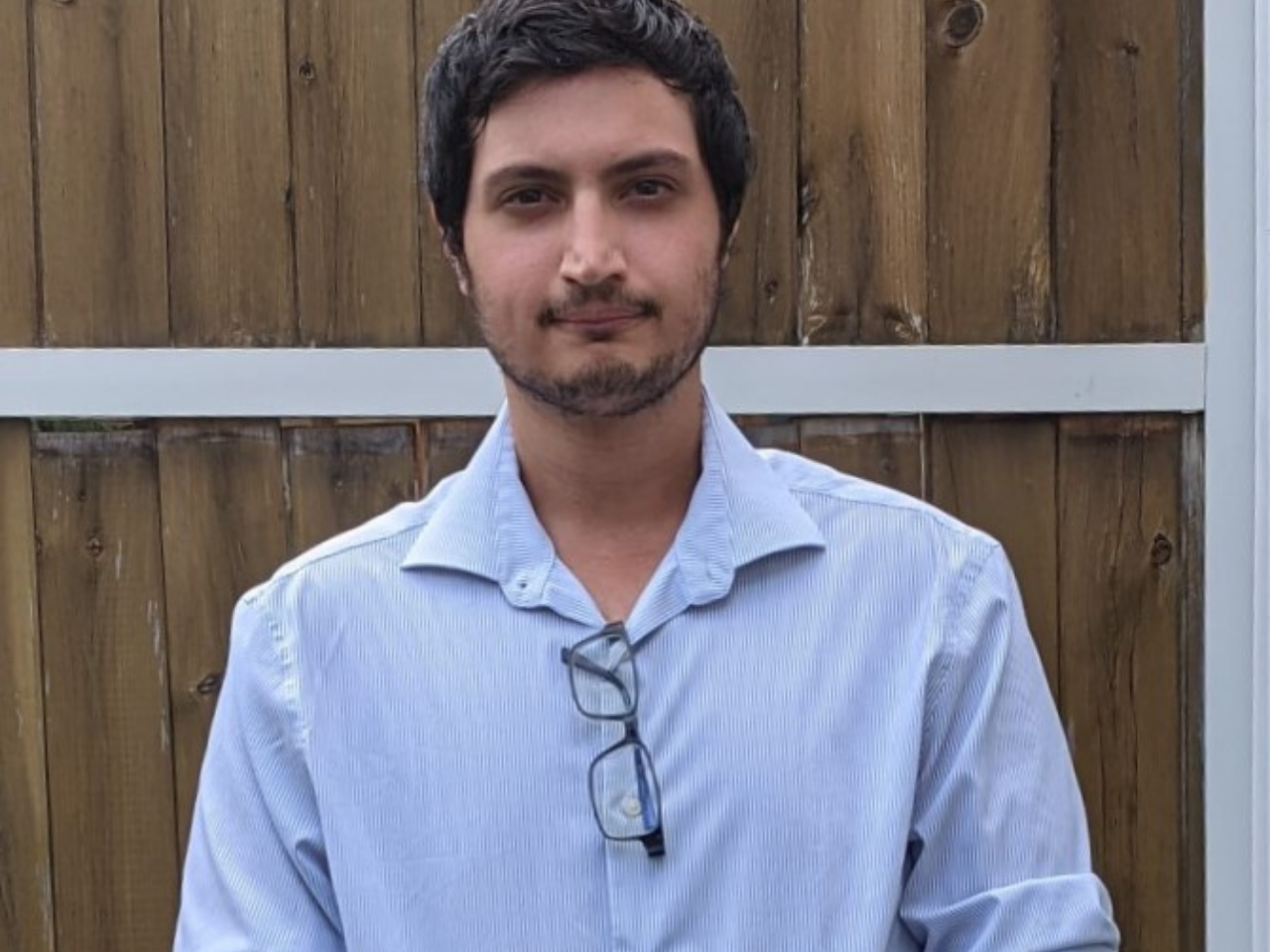
Kevin also developed models to estimate the volume of lesions in brain images using a modified Poisson regression method, which is used in statistics to model response variables. These models accurately identify the brain image with larger lesion volume. A possible application for this imaging method is in modelling the growth of brain lesions and tumors in patients. Despite their advantages, automated methods are not immune to error, and we are still far from trusting them for decision-making. Kevin proposed a statistical approach to measure uncertainty in standard segmentation models.
These projects were part of Kevin Raina’s thesis, which was nominated for a prize. His external examiner remarked that this research presents a considerable amount of new material for a MSc thesis. He published two papers in the Proceedings of the International Joint Conference on Biomedical Engineering Systems and Technologies (BIOSTEC) 2020 and published his third paper in BIOSTEC 2021.
Kevin is currently pursuing PhD studies with Prof. Schmah, in which he is developing statistical machine learning techniques for neuroimaging and heart datasets. He hopes to eventually develop techniques that can improve diagnosis and prognosis for Alzheimer’s and Amyotrophic lateral sclerosis (ALS) patients. He strongly believes that success is one attempt away from a failure. He advises prospective students to keep working hard, never give up, and build good connections with people.