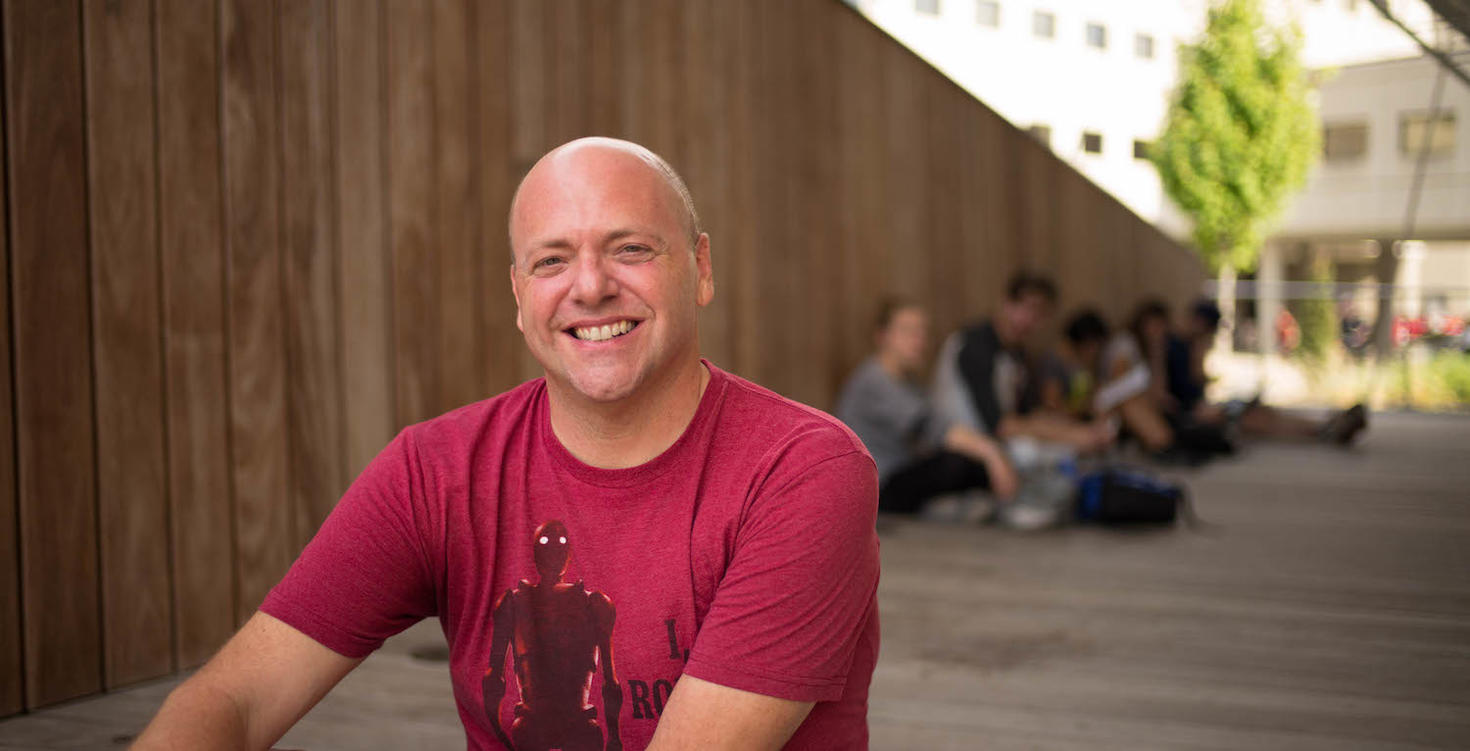
Le Sénat s'est engagé à s'informer, à discuter et à débattre de questions d'importance nationale telles que la façon de promouvoir l'innovation dans le domaine de la robotique tout en maximisant éthiquement les avantages sociaux de l'intégration robotique et de l'AI. Le professeur Kerr a appuyé sur la nécessité d'adopter une politique claire en vue du développement de l'IA.
Le professeur Ian Kerr, membre du Centre, est professeur titulaire à la Faculté de droit de l'Université d'Ottawa et titulaire de la Chaire de recherche du Canada en éthique, droit et technologie.
Les notes d’allocution (en anglais) sont reproduites ci-dessous.
Professeur Kerr a également publié une tribune dans le Ottawa Citizen (en anglais) en lien avec cette comparution.
Notes d'allocution (en anglais)
Imagine that you are looking for your Module L MRP. No, I don’t mean your Mobile Intelligent Robot Platform (MIRP) or some other robot. I mean your doctor. MRP stands for “most responsible physician” ... the practitioner most responsible for the in-hospital care of a particular patient. The MRP is responsible for writing orders, providing a care plan, obtaining consultations, and coordinating care.
Now imagine that you find your MRP. She is in the Module L corridor, saying goodbye to another patient. She tells you that she needs a few minutes to consult with Dr. Watson before your appointment. You are nervous. Today you are meant to receive your diagnosis and treatment plan for what is suspected to be some form of leukemia. It has been a difficult and unusual case, which is why Watson was brought in to help.
Watson, you learn at your appointment, is no doctor. It is an AI operating on top of an IBM supercomputer – a cognitive supercomputer designed to glean meaningful information from countless sources of structured and unstructured medical information. Watson is able to diagnose various forms of cancer with a success rate of 90%, significantly outperforming human doctors’ 50% success rate. Watson does so by scouring more than 20 million journal articles (an impossible task for human experts). Watson is one of many incredible breakthroughs in the field of artificial intelligence. We can expect countless other such breakthroughs in the near future.
Like other big data analytics AIs, Watson is essentially a sophisticated computer program. However, its design differs from conventional computing approaches in a way that raises unique legal and ethical challenges: it uses machine learning to excel at its diagnostic tasks. Watson is programmed to “ingest” vast quantities of unstructured medical data and related medical literature, and “learns” how to perform a diagnosis under the directed tutelage of human expert diagnosticians who train it using question-answer pairs and reinforcement learning. Once the human experts declare that Watson has reached a certain level of proficiency at the task, it is deemed expert enough to go into production. Just like human experts, Watson undergoes periodical “training updates,” reading the latest curated sets of information, and answering more questions under supervision designed to test its new knowledge. Compared to conventional computer programs, Watson is less like a tool, and more like a medical resident—always learning new medical information and occasionally making discoveries that would astonish an attending supervisor.
An important feature of machine learning-based AIs like Watson is their ability to outperform human experts, often by gleaning new insights or performing actions that surprise even their designers and expert trainers. This unpredictability by design, sometimes referred to as “emergent behaviour,” has been observed fairly consistently in widely reported public displays involving machine learning-based AIs, including Watson’s debut on the TV Game Show, Jeopardy!.
Meanwhile, back at the hospital, your MRP tells you that she finds herself in a rather existential state of mind – something you find ironic given that it is you who is in the life-or-death position.
Your MRP tells you that she finds herself in disagreement with Watson’s diagnosis. She reminds you that Watson has a 90% success rate compared to her 50% success rate in cases like this. She tells you she feels like Abraham on the mountain: she hears the voice of God telling her to sacrifice her only child, but that doesn’t sit right in her gut. She also tells you that her hospital is pushing her to follow the advice of the AI, concerned about the threat of a lawsuit if her gut is wrong. After all, the evidence seems to be on Watson’s side.
None of this sits right with you. Nor should it. You trust your doctor and your doctor does not trust the AI. But what if you trusted the AI against the advice of your doctor? Or, if your doctor trusted the AI, but you didn’t? What is the basis for such trust? How should we decide when to delegate important medical decisions to a machine and when to maintain meaningful human control? Is there a difference between the trust you perceive and the machine’s actual trustworthiness—a machine owned by the same company that helped facilitate Nazi genocide through generation and tabulation of punch cards based upon national census data in the 1930s? You are reminded of what your MRP explained earlier, namely that machine learning is unpredictable by design. Even though its record of past success is outstanding, it is unpredictable because it is designed to transcend its initial programming.
How do we decide when to delegate important medical decisions to a machine and when to maintain meaningful human control?
We can ask the exact same questions about when to let go of the wheel in the case of driverless cars.
And, we can ask the exact same question about when, if ever, to cede control to deadly military robots known as “lethal autonomous weapons.”
These difficult policy choices—which law makers must very quickly start to gain a much better appreciation of—are complicated by the way that law and policy might intervene on the problems themselves. As I already indicated, current medical malpractice law might actually spur the loss of human control when robots outperform humans. Hospitals, for example, might be forced to use robots instead of just doctors for treatment decisions, for surgeries and for other kinds of therapies because—not to do so—might one day soon fall below the standard of medical care required by law.
As a result, robots could start to displace doctors in certain areas much in the same way that society is concerned about technological unemployment in other realms. But it gets worse. In addition to the deskilling of human labour, in the case of Watson-type diagnosticians, we also risk a crisis in medical knowledge. We already stand at the precipice of machine-based medical decision-making that is so complex, that neither the machine’s programmers, nor the doctors who make use of them, are able to fully understand the decision-making process. Paradoxically, the machine is correct in its outcome—but its builders often don’t know why. If, in such cases, medical malpractice law pushes us to keep using such machines, we run the risk of creating an AI monoculture—databases will soon become full of medical decisions made exclusively by AIs without human interaction or intervention, leading to the possibility of suboptimal AI decision-making or path dependent errors of a sort that humans won’t easily be able to spot or fix. We will have relinquished control over medical knowledge and could possibly be the worse for it.
Of course, this may be avoided if we find the right ways of maintaining meaningful human control. But that would require changes to our laws, including the development of a regulatory structure for the use of AIs in the healthcare space.
Related challenges will occur in the automotive sector, which I am happy to discuss in greater detail in the question period.
Undoubtedly, the most crucial area where we need to set limits on what decisions can and cannot be delegated to AIs is International Humanitarian Law, and the policy questions relating to the development and use of lethal autonomous weapons. Here, we are talking about weapons that can sense, operate, target and kill without human intervention or oversight. To deploy these “killer robots” is to relinquish human control by delegating the kill decision to the machine itself.
Incredibly, Canada has not joined the host of other countries at the United Nations seeking to ban such weapons. In fact, Canada has not developed any clear policy position on the issue. As such, Canada is missing an important opportunity for global leadership on the international stage. Leadership that is necessary to avoid mass atrocity and destruction on an international scale.
There is a deep conundrum in all of this. The same underlying AI can be engines of creation or engines of destruction. Given Canada’s potential for leadership in the development of AI, perhaps our greatest challenge in the coming decades will be how to ensure beneficial AI.
Those are my seven minutes. We have lots to talk about during the discussion period.